MDS Newsletter #51

Hey folks,
The very first episode of the Modern Data Show is out now! We are available on Spotify, Google Podcast, YouTube and Amazon music. Do give us a follow!
The Modern Data Show
S01 E01: Understanding the data platform at Canva with Greg Roodt
Managing ETL processes, data infrastructure, and data teams is already an arduous task, imagine doing all this in a very data-intensive organisation! In this episode, Greg takes us through how he and his team have kept things rolling at Canva. Greg walks us through what data platforms they use at Canva, ETL pipelines, data team structure, and much more.
Listen Now👇
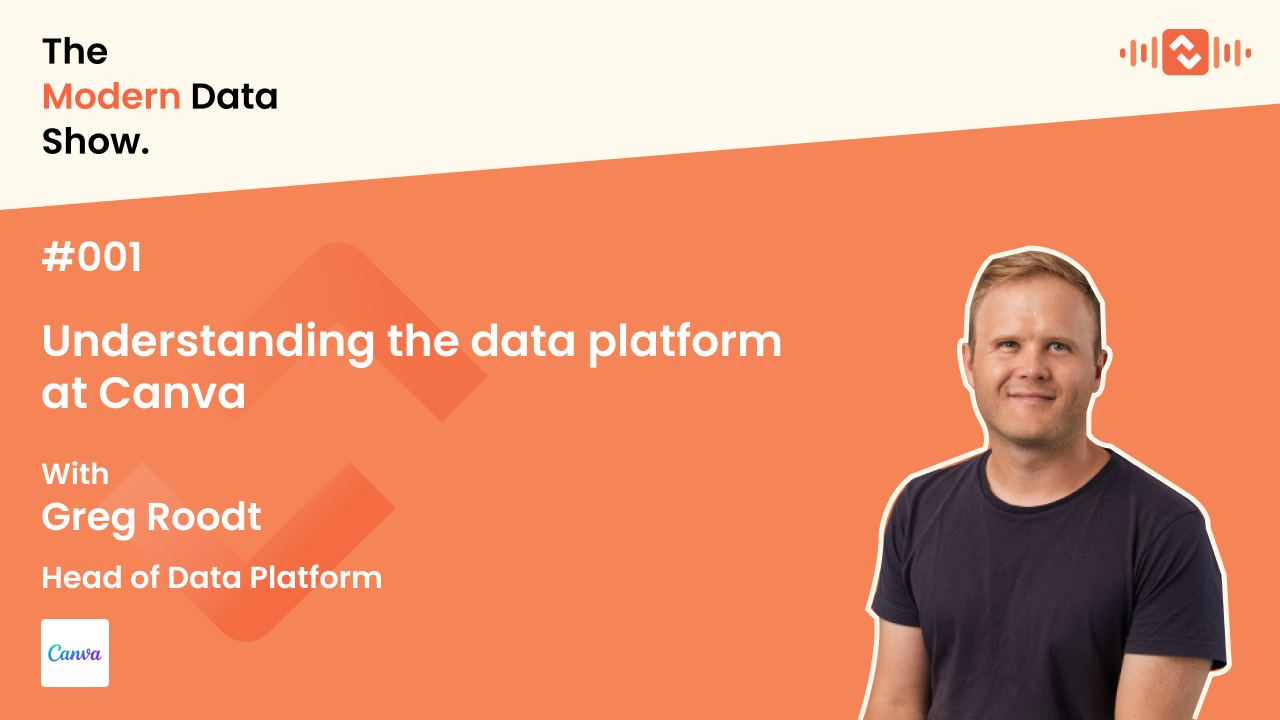
Featured tools of the week
- Coginiti is a collaborative intelligence company that empowers everyone to get consistent answers fast to any business question. Cogniti's collaborative intelligence platform provides a unique workspace that empowers the entire organization to build, share and reuse analytics. By making quality data widely available and focusing on outcomes over pre-defined output, everyone is freed up to explore and experiment to answer business questions.
Coginiti has raised a total of $4M in funding over 1 round. This was a Seed round raised on Mar 12, 2022. - Shipyard is an orchestration platform for data engineers to build a solid data infrastructure from the get-go by connecting data tools and processes and streamlining data workflows. Shipyard offers low-code templates that are configured using a visual interface, replacing the need to write code to build data workflows while enabling data engineers to get their work into production faster.
Featured data stack of the week
- You Need A Budget teaches employees a whole new way of thinking about their money.  Your money doesn't have to be messy. Get a handle on your finances with YNAB—a proven method and budgeting app that gives you real results.
Here's how their data stack looks like
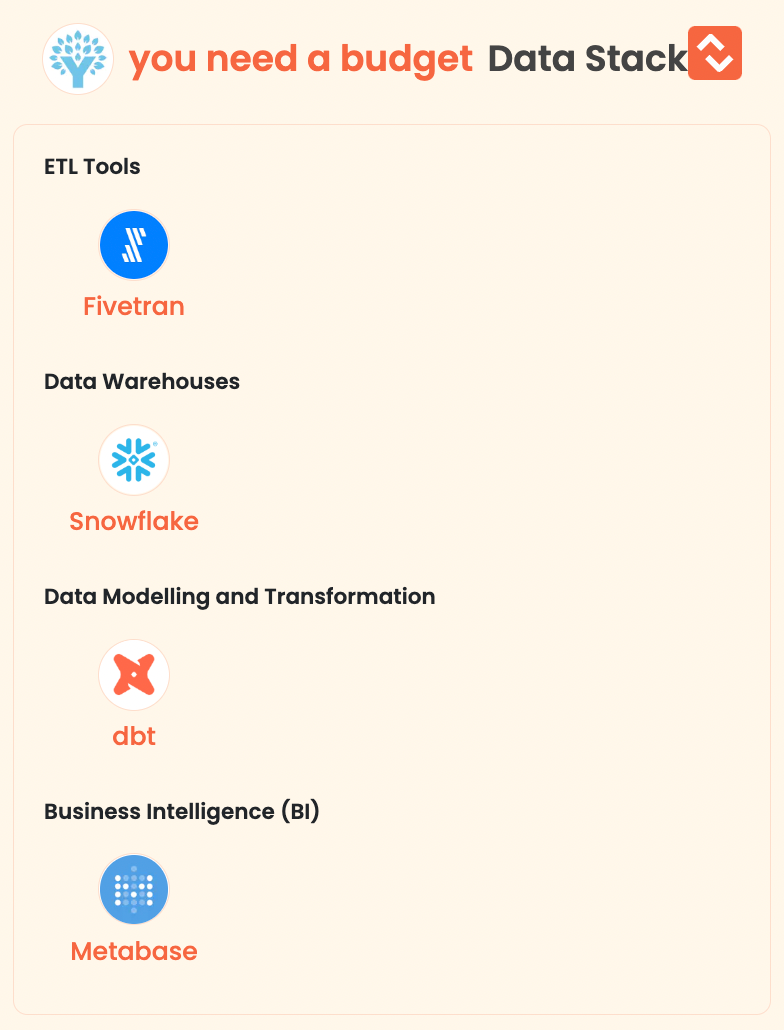
Good reads and resources
- Organizations need to deliberately create data: 'Data as oil' is an extensively used metaphor and its impact can be gauged by how we currently use and work with data. Every business is heavily dependent on the data provided to them by other organisations. The world of data scientists is bounded by the data they can 'extract' and sometimes they have to abandon developing a particular feature because lack of the desired data. Source data systems are finite: they have a certain amount of data with a certain associated scope. Data quality has become an even bigger problem in the data space, there's not much an organisation can do to fix the quality of the source data. So if data extraction has so many problems, what's an alternative? Yali Sassoon explores the alternative to data extraction which is 'data creation. He has made a compelling case why organisations should invest in creating better data - and that investment can directly drive value and competitive advantage.
To be fair, a huge amount of value has been unlocked by taking that data, bringing it together in a cloud data warehouse/lakehouse, and then building intelligence and activation on top of it. But so much more can be accomplished if organizations look beyond the data that they happen to have today, and start to deliberately create better data to power their data apps for tomorrow - Yali Sassoon
- The Great Data Paradox - Governance vs. Self-Service: With the rise of modern data tooling ecosystem no one back in 1993, when OLAP cubes were invented, would have imagined the computing power amassed by database systems. Traditional BI, which required specially built applications to operate between the business user and the data, gave way to Self-Service BI. But the rise of self-serve BI tools not only made data governance a challenge but also gave rise to the great data paradox - governance vs. self-service. Josh Berry in this blog has discussed why the data industry needs self-service with guardrails and how to deploy standardized, self-service metrics within your organization.
In summary, we see that OLAP provided the governance we wanted, while BI gave us the self-service we needed. The paradox is that these two aspects of data management appear to be at odds with one another. - Josh Berry
Journal
- How important is data lineage in terms of data observability?: There's a lot of confusion around data lineage, data observability and interdependencies. Data Lineage is one of the most discussed topics today and so is data observability. The article by Mona Rakibe covers the applicability of data lineage in Data Observability. She has explored in detail about data lienage, data observability and how data lineage is used in data observability.
If you have an interesting blog that you would like us to share with the data community, submit it here.
Upcoming data events and summits
- Atlan has introduced a 'Masterclass Series' – a 5-part series on building a DataOps culture. The first masterclass is on 'Applying a Data Product Mindset to Design a DataOps Program for Diverse Data Users' on 15th September at 2:30 PM ET.
Join the event to learn how to apply a data product mindset to understand the current behaviours, beliefs, and blockers of your data users. - Economist Impact is organising an event on 'The data dividend: unlocking data-driven insight and innovation' on September 22, 2022.
Join the event to listen to discussions about how data can be better harnessed to fuel innovation and unlock the potential of artificial intelligence (AI). You can also watch the live stream of the event. Bookmark here.
MDS Jobs
- Mammoth Growth is hiring an 'Analytics Engineering Lead'
Location: Remote
Data stack:dbt, Snowflake, BigQuery, Redshift
Apply here - Sigma Computing is hiring a 'Modern Data Stack Solution Engineer'
Location: USA
Data stack: Snowflake, databricks, dbt, fivetran, & Sigma
Apply here. - Vercel is hiring a 'Analytics Engineer'
Location: US / Canada
Data stack: Fivetran,dbt, Superset
Apply here
🔥 on Twitter
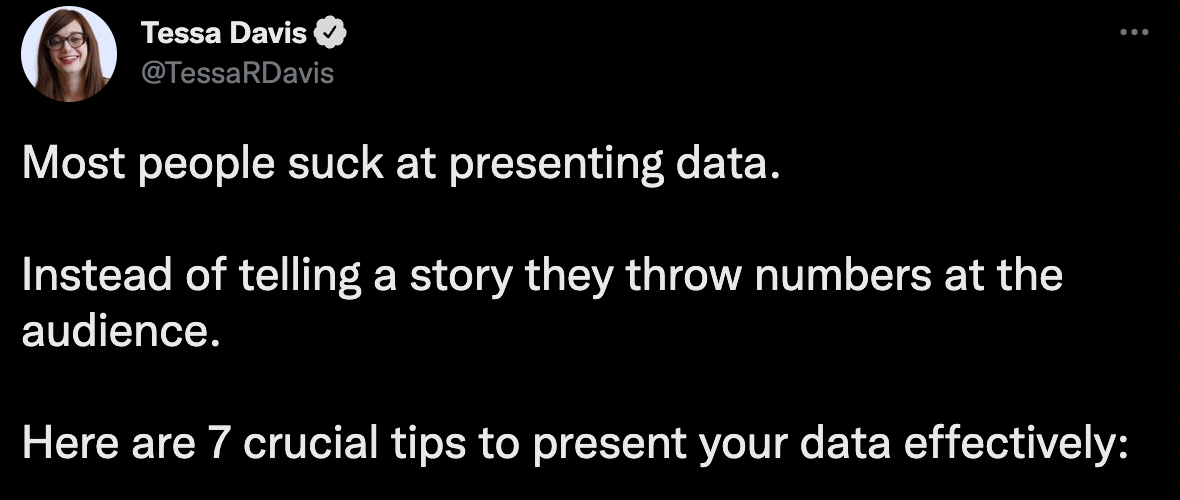
Just for fun 😃
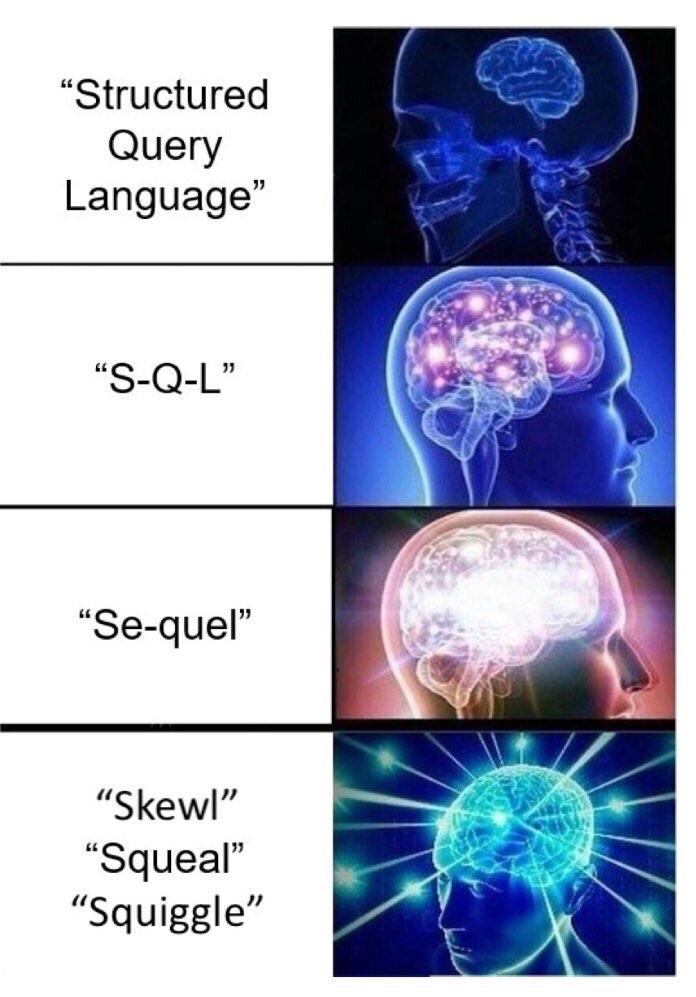
Subscribe to our Newsletter, Follow us on Twitter and LinkedIn, and never miss data updates again.
What do you think about our weekly Newsletter?
Love it | It's great | Â Good | Okay-ish | Meh
If you have any suggestions, want us to feature an article, or list a data engineering job, hit us up! We would love to include it in our next edition😎
About Moderndatastack.xyz‌‌ - We're building a platform to bring together people in the data community to learn everything about building and operating a Modern Data Stack. It's pretty cool - do check it out :)